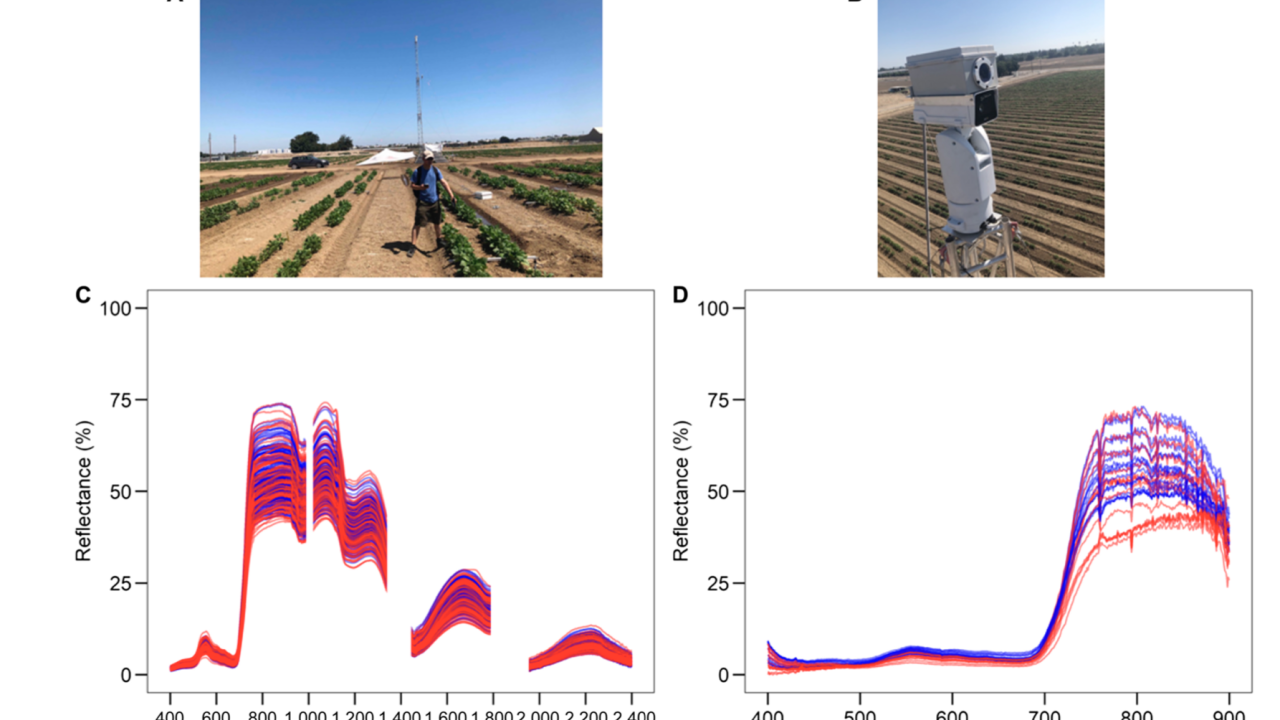
New paper led by Chris Wong predicting stomatal conductance in beans using hyperspectral data and machine learning
Proximal remote sensing offers a powerful tool for high-throughput phenotyping of plants for assessing stress response. Bean plants, an important legume for human consumption, are often grown in regions with limited rainfall and irrigation and are therefore bred to further enhance drought tolerance. We assessed physiological (stomatal conductance and predawn and midday leaf water potential) and ground- and tower-based hyperspectral remote sensing (400 to 2,400 nm and 400 to 900 nm, respectively) measurements to evaluate drought response in 12 common bean and 4 tepary bean genotypes across 3 field campaigns (1 predrought and 2 post-drought). Hyperspectral data in partial least squares regression models predicted these physiological traits (R2 = 0.20 to 0.55; root mean square percent error 16% to 31%). Furthermore, ground-based partial least squares regression models successfully ranked genotypic drought responses similar to the physiologically based ranks. This study demonstrates applications of high-resolution hyperspectral remote sensing for predicting plant traits and phenotyping drought response across genotypes for vegetation monitoring and breeding population screening.
Wong, C. Y., Gilbert, M. E., Pierce, M. A., Parker, T. A., Palkovic, A., Gepts, P., ... & Buckley, T. N. 2023. Hyperspectral remote sensing for phenotyping physiological drought response of common & tepary bean. Plant Phenomics. (5), 0021. https://doi.org/10.34133/plantphenomics.0021