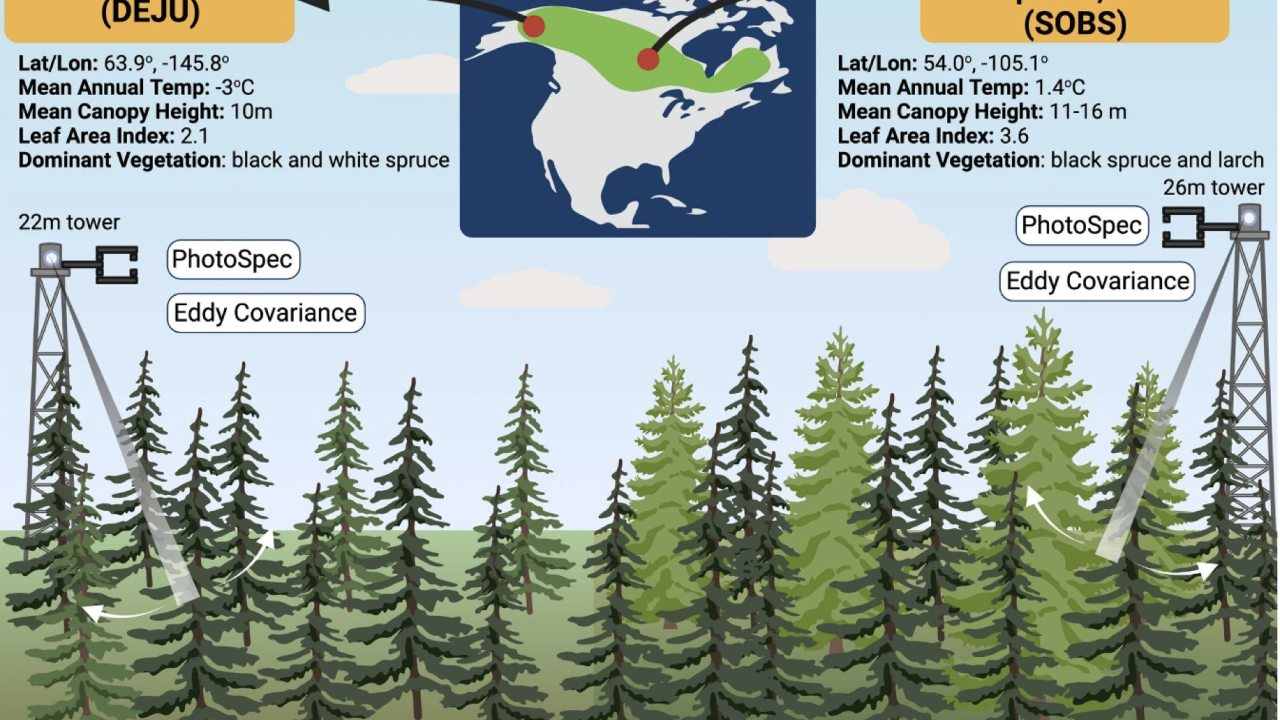
'Forests for Forests' paper published
Quick Summary
- Using Random Forest Models to predict Evergreen Photosynthesis using tower based remote sensing
Remote sensing is a powerful tool for understanding and scaling measurements of plant carbon uptake via photosynthesis, gross primary productivity (GPP), across space and time. The success of remote sensing measurements can be attributed to their ability to capture valuable information on plant structure (physical) and function (physiological), both of which impact GPP. However, no single remote sensing measure provides a universal constraint on GPP and the relationships between remote sensing measurements and GPP are often site specific, thereby limiting broader usefulness and neglecting important nuances in these signals. Improvements must be made in how we connect remotely sensed measurements to GPP, particularly in boreal ecosystems which have been traditionally challenging to study with remote sensing. In this paper we improve GPP prediction by using random forest models as a quantitative framework that incorporates physical and physiological information provided by solar-induced fluorescence (SIF) and vegetation indices (VIs). We analyze 2.5 years of tower-based remote sensing data (SIF and VIs) across two field locations at the northern and southern ends of the North American boreal forest. We find (a) remotely sensed products contain information relevant for understanding GPP dynamics, (b) random forest models capture quantitative SIF, GPP, and light availability relationships, and (c) combining SIF and VIs in a random forest model outperforms traditional parameterizations of GPP based on SIF alone. Our new method for predicting GPP based on SIF and VIs improves our ability to quantify terrestrial carbon exchange in boreal ecosystems and has the potential for applications in other biomes.
Pierrat, Z. A., Bortnik, J., Johnson, B., Barr, A. G., Magney, T. S., Bowling, D., ... & Stutz, J. (2022). Forests for Forests: Combining vegetation indices with solar-induced chlorophyll fluorescence in random forest models improves gross primary productivity prediction in the Boreal Forest. Environmental Research Letters.
https://iopscience.iop.org/article/10.1088/1748-9326/aca5a0/meta